Credit Card Fraud Detection Architecture
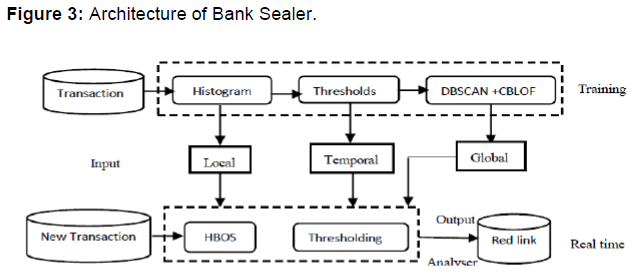
Enormous data is processed every day and the model build must be fast enough to respond to the scam in time.
Credit card fraud detection architecture. Nowadays credit card fraud detection is of great importance to finan cial institutions. Thus when i came across this data set on kaggle dealing with credit card fraud detection i was immediately hooked. Intercepting of mails containing newly issued cards copying and replicating of card information through skimmers or gathering. If you are interested in studying past trends and training machines to learn with time how to define scenarios identify and label events or predict a value in the present or future data science is of the essence.
The data set has 31 features 28 of which have been anonymized and are labeled v1 through v28. Nbknn for fraud detection of credit card. Credit card fraud detection issues display a mods operand and act as a model of carrying out a fraud credit card transaction. Experimental results illustrate that both classifiers work differently for the same dataset.
The challenge is to recognize fraudulent credit card transactions so that the customers of credit card companies are not charged for items that they did not purchase. This article presents an automated credit card fraud detection sys tem based on the neural network technology. Fraudsters usually get hold of card information in a variety of ways. Main challenges involved in credit card fraud detection are.
Credit card fraud involves illegal use of card or card information without the knowledge of the owner and hence is an act of criminal deception. By exactly pinpointing those fraudulent transactions this particular model is then utilized for identifying if any new transaction being carried out is a fraud or not through ai enabled algorithms and applications. The remaining three features are the time and the amount of the transaction as well as whether that transaction was fraudulent or not. Here i will be mainly focusing on credit card fraud detection and talk about the techniques approaches and lessons learnt based on our experience in building a machine learning application for.